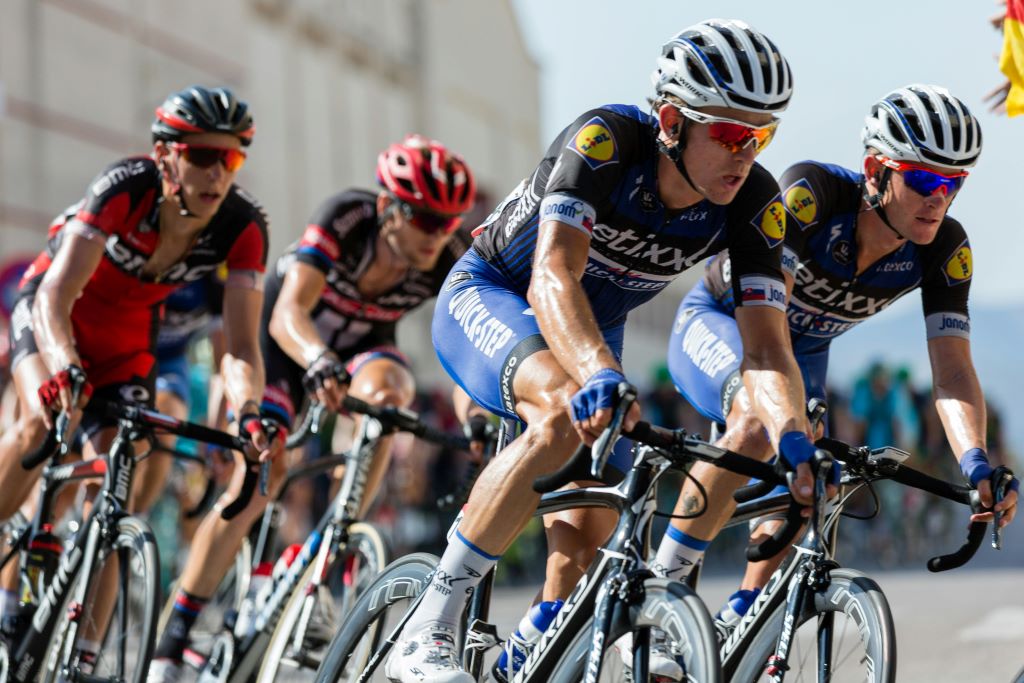
DeepSeek-R1 represents a significant advancement in the field of artificial intelligence, particularly in enhancing the reasoning capabilities of Large Language Models (LLMs). Developed by DeepSeek, this model introduces innovative training methodologies that reduce reliance on supervised fine-tuning, thereby lowering development costs and democratizing access to advanced AI technologies.
Key Innovations in DeepSeek-R1
- Reinforcement Learning-Centric Training: DeepSeek-R1’s training regimen emphasizes reinforcement learning (RL) over traditional supervised fine-tuning. By employing a rule-based RL approach, the model autonomously refines its reasoning abilities, minimizing the need for extensive human-labeled datasets.
- Group Relative Policy Optimization (GRPO): A notable innovation in DeepSeek-R1’s development is the use of GRPO, an in-house RL method. GRPO samples multiple outputs for a given input and calculates rewards based on predefined rules, such as accuracy and format adherence. This technique enables the model to learn from its own outputs, fostering self-improvement.
- Open-Source Commitment: Aligning with DeepSeek’s mission to make AI research accessible, DeepSeek-R1 is released under the MIT License. This open-source approach invites collaboration and accelerates advancements in AI research and application.
Implications for the AI Landscape
The introduction of DeepSeek-R1 has garnered significant attention within the AI community and beyond:
- Performance and Efficiency: DeepSeek-R1 rivals the performance of established models like OpenAI’s o1 across various tasks, including mathematical reasoning and code generation. Remarkably, it achieves this at a fraction of the development cost, challenging the notion that high-performing AI models require substantial financial investment.
- Global Impact: The model’s success has sparked discussions about China’s rapid advancements in AI, highlighting the country’s growing competitiveness in the global AI landscape.
Conclusion
DeepSeek-R1 exemplifies a pivotal shift in AI development, demonstrating that innovative training methodologies can produce high-performing models without heavy reliance on supervised fine-tuning. Its open-source nature not only democratizes access to advanced AI but also fosters a collaborative environment for future research and development.
Reference
DeepSeek-R1: Incentivizing Reasoning Capability in LLMs via
Reinforcement Learning 2501.12948